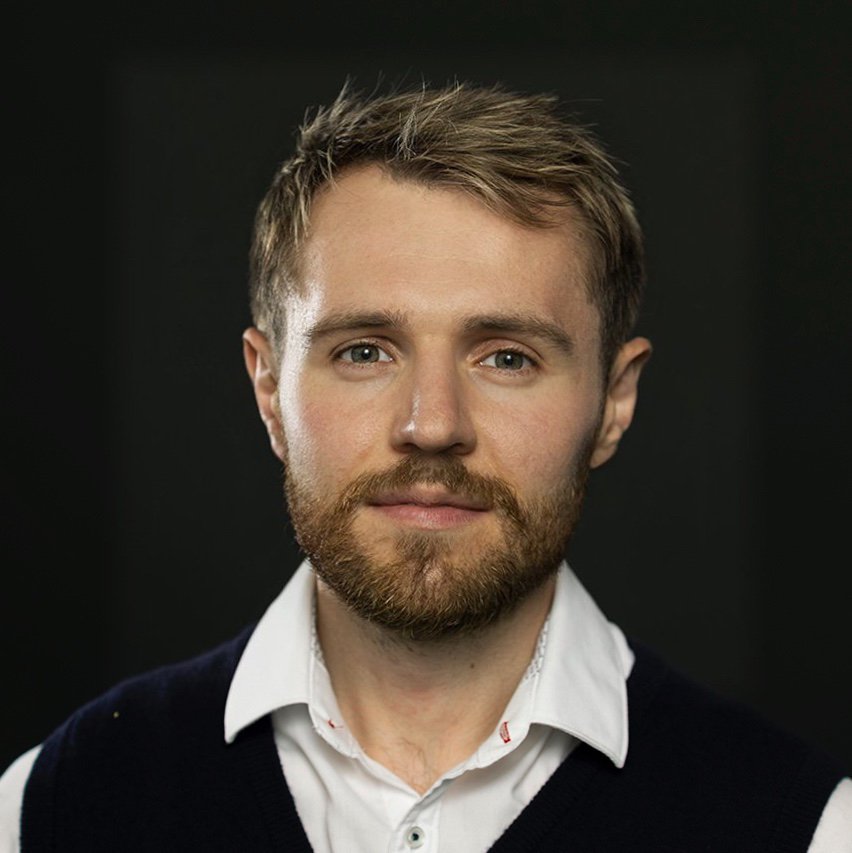
I'm Arthur Bražinskas, a Research Scientist at Google DeepMind, where I co-lead the user signal efforts for Gemini. I founded this initiative and helped shape the organization’s vision for using real-world user feedback to evaluate and improve model quality. My work focuses on the post-training phase of model development, with a particular emphasis on Reinforcement Learning from Human Feedback (RLHF) to better align our models with human preferences and behavior.
I received a Ph.D. degree from the University of Edinburgh in 2022. I was fortunate to be supervised by Ivan Titov and Mirella Lapata. My thesis was on opinion summarization with a focus on low-resource learning. In this setting, annotated datasets are scarce, and models are fine-tuned on a handful of annotated samples in a sample-efficient manner. Additionally, I was the ILCC representative, helping to establish an inclusive community within the group.
On the machine learning side, I’m interested in Bayesian approaches that model data in terms of random/stochastic variables. These models can naturally capture uncertainty and represent information that is not directly observable in datasets. For training, my methods of choice are variational auto-encoders (VAE) and reinforcement learning.
Before the Ph.D., I graduated (MSc. \w distinction) in artificial intelligence from the University of Amsterdam, Netherlands, where I specialized in theoretical machine learning and natural language processing. Before starting my Ph.D., I worked on machine learning modeling at Elsevier, Amazon, and Zalando. I also interned at Amazon under the supervision of R. Nallapati, M. Bansal, M. Dreyer.